Table Of Content
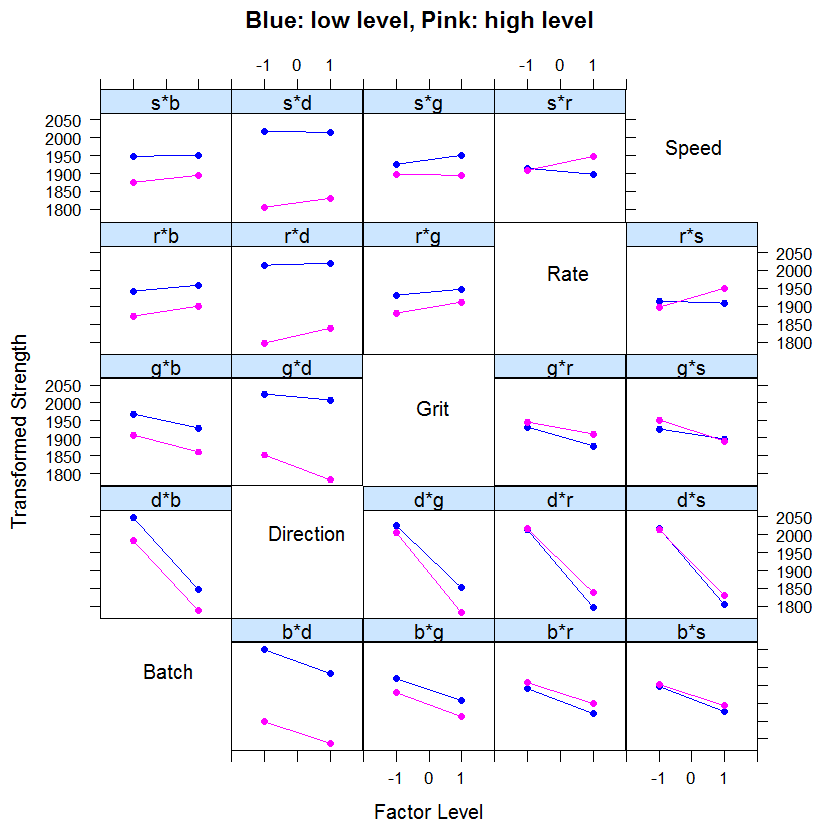
Instead, it involves measuring several variables, often both categorical and quantitative, and then assessing the statistical relationships among them. These included health, knowledge of heart attack risk factors, and beliefs about their own risk of having a heart attack. They found that more optimistic participants were healthier (e.g., they exercised more and had lower blood pressure), knew about heart attack risk factors, and correctly believed their own risk to be lower than that of their peers. Another common approach to including multiple dependent variables is to operationalize and measure the same construct, or closely related ones, in different ways.
Factorial Designs¶
In the 2 × 3 example, for instance, the pattern of the A column follows the pattern of the levels of factor A, indicated by the first component of each cell. Regression analysis is used to model the relationship between two or more variables in order to determine the strength and direction of the relationship. There are several types of regression analysis, including linear regression, logistic regression, and multiple regression.
2.2. Factorial Designs¶
You would find these types of designs used where k is very large or the process, for instance, is very expensive or takes a long time to run. In these cases, for the purpose of saving time or money, we want to run a screening experiment with as few observations as possible. When we introduced this topic we wouldn't have dreamed of running an experiment with only one observation. As a matter of fact, the general rule of thumb is that you would have at least two replicates. This would be a minimum in order to get an estimate of variation - but when we are in a tight situation, we might not be able to afford this due to time or expense.
IX. Chapter 9: Factorial Designs
Create your experimental design with a simple Python command by Tirthajyoti Sarkar - Towards Data Science
Create your experimental design with a simple Python command by Tirthajyoti Sarkar.
Posted: Wed, 04 Jul 2018 02:31:34 GMT [source]
SuperGym currently categorizes her clients into 4 body types to help plan for the best possible program. Since this is a first order, linear model, the coefficients can be combined with the operating parameters to determine equations. Half Normal Plots for wt% methanol in biodiesel and number of theoretical stages are shown below. The next step is selecting which terms will be analyzed for the responses.
Two-level factorial experiments
Two-level fractional factorial designs provide efficient experiments to screen a moderate number of factors when many of the factorial effects are assumed to be unimportant (sparsity) and when an effect hierarchy can be assumed. They are simple to design and analyze, while providing information that can be used to inform more detailed follow-up experiments using only the factors found to be important. More details on full and fractional factorial designs can be found in ref. 4. Factorial designs can pose challenges, but they offer important advantages that can offset such challenges.
However, it is important to make sure the individual dependent variables are correlated with each other by computing an internal consistency measure such as Cronbach’s \(\alpha\). If they are not correlated with each other, then it does not make sense to combine them into a measure of a single construct. If they have poor internal consistency, then they should be treated as separate dependent variables. This main total effect value for each variable or variable combination will be some value that signifies the relationship between the output and the variable. For instance, if your value is positive, then there is a positive relationship between the variable and the output (i.e. as you increase the variable, the output increases as well).
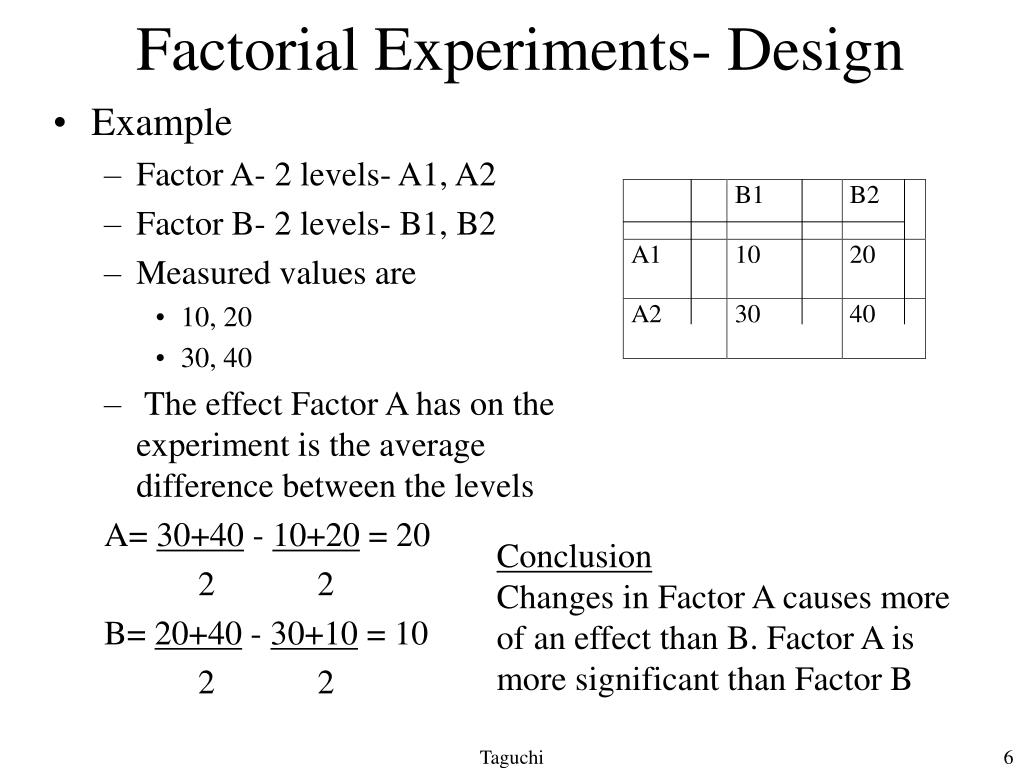
Other useful exploratory analysis tools for factorial experiments include main effects plots, interaction plots, Pareto plots, and a normal probability plot of the estimated effects. When we use a 2×2 factorial design, we often graph the means to gain a better understanding of the effects that the independent variables have on the dependent variable. Again, because neither independent variable in this example was manipulated, it is a cross-sectional study rather than an experiment. The advantage of multiple regression is that it can show whether an independent variable makes a contribution to a dependent variable over and above the contributions made by other independent variables.
In many studies, the primary research question is about an interaction. The study by Brown and her colleagues was inspired by the idea that people with hypochondriasis are especially attentive to any negative health-related information. This led to the hypothesis that people high in hypochondriasis would recall negative health-related words more accurately than people low in hypochondriasis but recall non-health-related words about the same as people low in hypochondriasis. The effect of one independent variable can depend on the level of the other in several different ways. By doing this, psychologists can see if changing the independent variable results in some type of change in the dependent variable. Like Pareto plots, Half Normal plots show which factors have significant effects on the responses.
Results from this study suggested that participants high in hypochondriasis were better than those low in hypochondriasis at recalling the health-related words, but that they were no better at recalling the non-health-related words. In many factorial designs, one of the independent variables is a non-manipulated independent variable. Some were negative health-related words (e.g., tumor, coronary), and others were not health related (e.g., election, geometry). In a between-subjects factorial design, all of the independent variables are manipulated between subjects. For example, all participants could be tested either while using a cell phone or while not using a cell phone and either during the day or during the night. In a within-subjects factorial design, all of the independent variables are manipulated within subjects.
These measures may include reaction time tasks, cognitive tests, or other types of computer-based assessments. In summary, the authors’ conclusions about the efficacy of light flashes are likely to be correct (within the limits of self-reported outcomes, which they note). That said, when designing studies that address more than 1 question, when possible, one should consider the potential advantages of unconfounding those factors by conducting a factorial experiment. This is one approach to assume that some interactions are not important and use this to test lower order terms of the model and finally come up with a model that is more focused.
Research Methods in Psychology Copyright © 2019 by Rajiv S. Jhangiani, I-Chant A. Chiang, Carrie Cuttler, & Dana C. Leighton is licensed under a Creative Commons Attribution-NonCommercial-ShareAlike 4.0 International License, except where otherwise noted. This website is using a security service to protect itself from online attacks. There are several actions that could trigger this block including submitting a certain word or phrase, a SQL command or malformed data.
The material found on this site is for informational purposes only and is not intended to be used for diagnosis or treatment purposes. So, the researchers decided to look at how the amount of sleep and caffeine influence test performance. “It is true that when two manipulations are operating simultaneously, it is impossible to disentangle their effects completely,” explain authors Breckler, Olson, and Wiggins in their book Social Psychology Alive. You have been employed by SuperGym, a local personal training gym, who want an engineer's perspective on how to offer the best plans to their clients.
Frank Yates created an algorithm to easily find the total factorial effects in a 2n factorial that is easily programmable in Excel. While this algorithm is fairly straightforward, it is also quite tedious and is limited to 2n factorial designs. Thus, modern technology has allowed for this analysis to be done using statistical software programs through regression. By the traditional experimentation, each experiment would have to be isolated separately to fully find the effect on B. Note that only four experiments were required in factorial designs to solve for the eight values in A and B. Suppose you have two variables \(A\) and \(B\) and each have two levels a1, a2 and b1, b2.
The other approach is a weighted analysis, where you weight the observations according to the inverse of their variance. There are situations where you have unequal variation for maybe a known reason or unknown reason, but if you have repeated observations and you can get weights, then you can do a weighted analysis. For those of you who have studied heteroscedastic variance patterns in regression models you should be thinking about possible transformations. There is a gap in the histogram of other residuals but it doesn't seem to be a big problem.
When there are two independent variables, each with two levels, there are four total conditions that can be tested. Thus, to the extent that two ICs affect coping execution or withdrawal severity, their co-occurrence in the experiment could reduce estimates of their main effects via negative interaction. One might think of this as interventions “competing” for a limit subset of participants who are actually capable of change or improvement; in a sense this subsample would be spread across multiple active intervention components. In a factorial design, participants are randomly assigned to one of several groups, each of which receives a different combination of two or more independent variables.
No comments:
Post a Comment